What is Marketing Mix Modeling (MMM)?
Learn everything you need to know about optimizing your ad spend with marketing mix modeling.
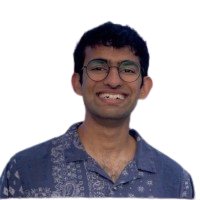
Tejas Manohar
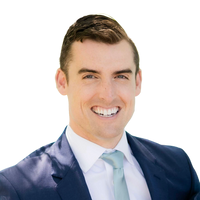
Nate Wardwell
November 6, 2023
9 minutes
Marketing Mix Modeling Use Cases
MMM can be used for any type of analytics and decision-making that attribution powers. Common use cases specifically for MMM include:
- Optimize budget allocation. MMM indicates which channels provide the best return on investment (ROI), helping you optimize spend across channels like TV, radio, and online advertising to accomplish your goals.
- Align marketing strategies across teams. MMM provides a common language and framework to evaluate marketing channels. This allows disparate teams at enterprise organizations to collaborate on the best campaigns and ways to distribute budget and effort.
- Predict future outcomes and test new strategies. MMM predicts future outcomes so you can adjust your marketing spend and try alternative variations in a theoretical environment.
- Adjust strategies for seasonality. MMM clearly outlines the impacts of seasonality on your target outcomes so you can predict future metrics and adjust your inputs accordingly to meet your goals.
Marketing Mix Modeling vs. Digital Tracking
Digital tracking directly measures individual customer interactions before conversion to attribute outcomes to each touchpoint. Last-touch attribution gives full “credit” for the conversion to the last interaction a customer had before converting, while first-touch attribution gives full credit to the first customer interaction. More sophisticated attribution models distribute credit differently across multiple customer touchpoints, such as multi-touch attribution (MTA), linear attribution, time-decay attribution, and u-shaped attribution.
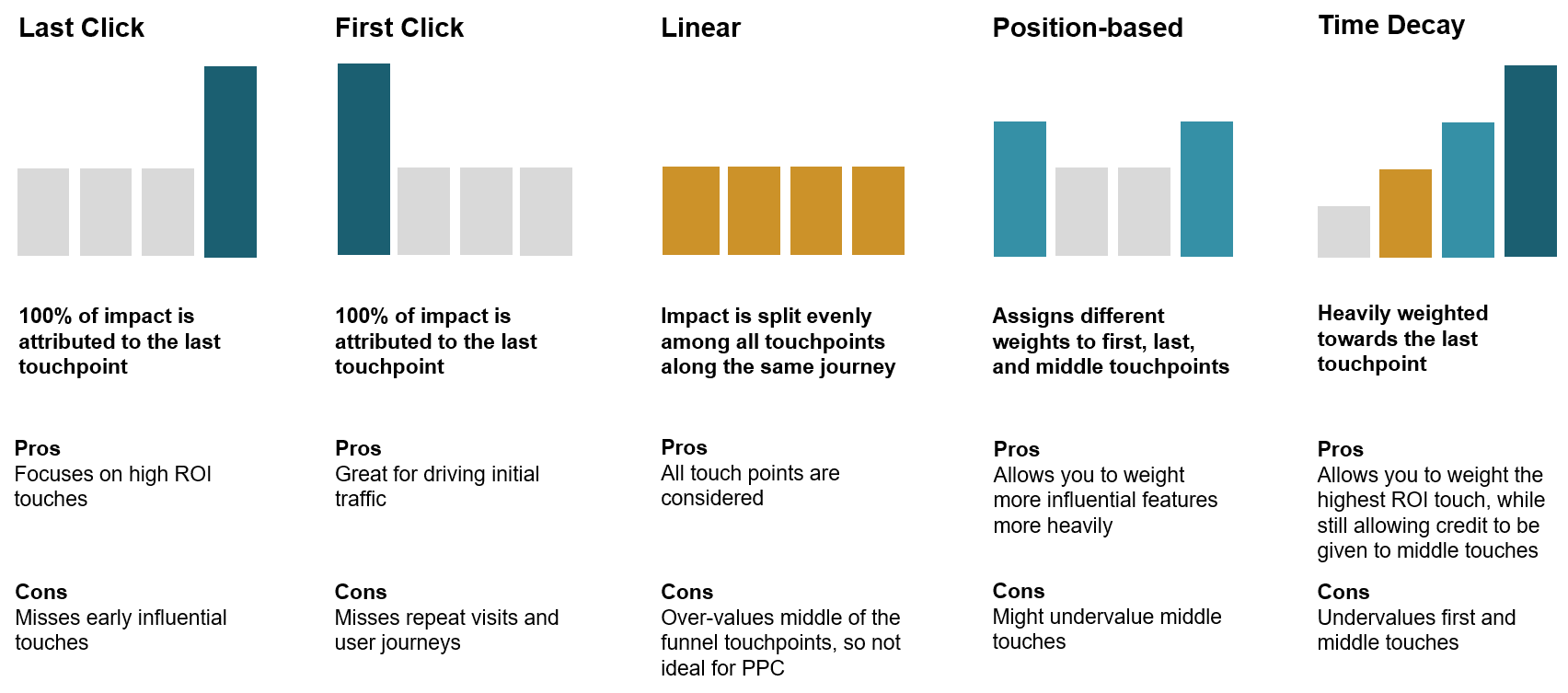
Source: https://www.linkedin.com/pulse/its-attribution-baby-mmm-vs-mta-sascha-st%C3%BCrze/
Attribution models are a powerful way to understand causality from digital touchpoints such as paid search and social media platforms and are very accurate because they directly measure consumer behavior. This utility and directness have made attribution the default mode for decision-making in digital marketing.
Data-collection headwinds are now plaguing attribution models. Increased privacy regulations and the decline of third-party cookies have already reduced the effectiveness of traditional digital attribution. Further adaptions by major tech players like Google and Apple to moderate user privacy and even restrict standard data-tracking techniques like UTM parameters will further hobble digital attribution.
Unlike attribution models, MMM does not rely on directly tracking individual users through their touchpoints before conversion. Instead, MMM analyzes aggregate historical data from sales and marketing channels. MMM is, therefore, immune to the changing privacy landscape online and has grown in popularity in recent years as a result. MMM is also uniquely focused on the incrementality of each marketing dollar spent, while digital tracking models focus more on the collection of touchpoints that lead to individual conversions.
Limitations to Marketing Mix Modeling
Marketing mix modeling can be highly effective for optimizing marketing budgets, but it does have several limitations to consider:
- MMM requires the correct data. If you haven’t already collected historical data on channel inputs and dependent variable outputs, you’ll have to start collecting more data and wait for maturity until you can build an adequate model.
- MMM doesn’t capture individual interactions. MMM makes overall predictions about the impact of channel spending on outcomes, but it is far less granular and accurate on a per-user basis than digital attribution models.
- MMM requires statistical sophistication. MMM is complex to execute. You must hire analysts or work with an external team or tool. No matter how you approach it, MMM has a high built-in cost.
- MMM can be imperfect. The quality of your model can vary widely based on your inputs and the effort you put into compiling it. It’s also important to note that MMM models can also fall short when measuring the long-term effects of channels and will not be a good predictor of changes in consumer behavior or the overall market.
Tools and Software for Marketing Mix Modeling
While MMM can be performed by any (sophisticated) analyst with languages like Python or R, several open-source solutions can act as an accelerator for you:
- Robyn is a popular open-source MMM package produced by Meta (Facebook) that leverages machine learning
- LightweightMMM is Google’s open-source MMM package that uses Bayesian methods.
- PyMC Marketing is PyMC Lab’s open-source MMM solution.
There are also an increasing number of paid vendors that offer MMM solutions:
- Rockerbox allows teams to upload their data and then offers a variety of attribution models for marketing, including MMM.
- Recast is a fully-featured MMM platform with an advanced statistical modeling framework and robust tools for marketers doing planning and optimization.
- Paramark solves for both MMM and incrementality testing across both B2B and B2C companies.
- Finally, various full-service marketing partners and service agencies will also perform MMM, such as Media.Monks, Tredence, Blend360, and Artefact.
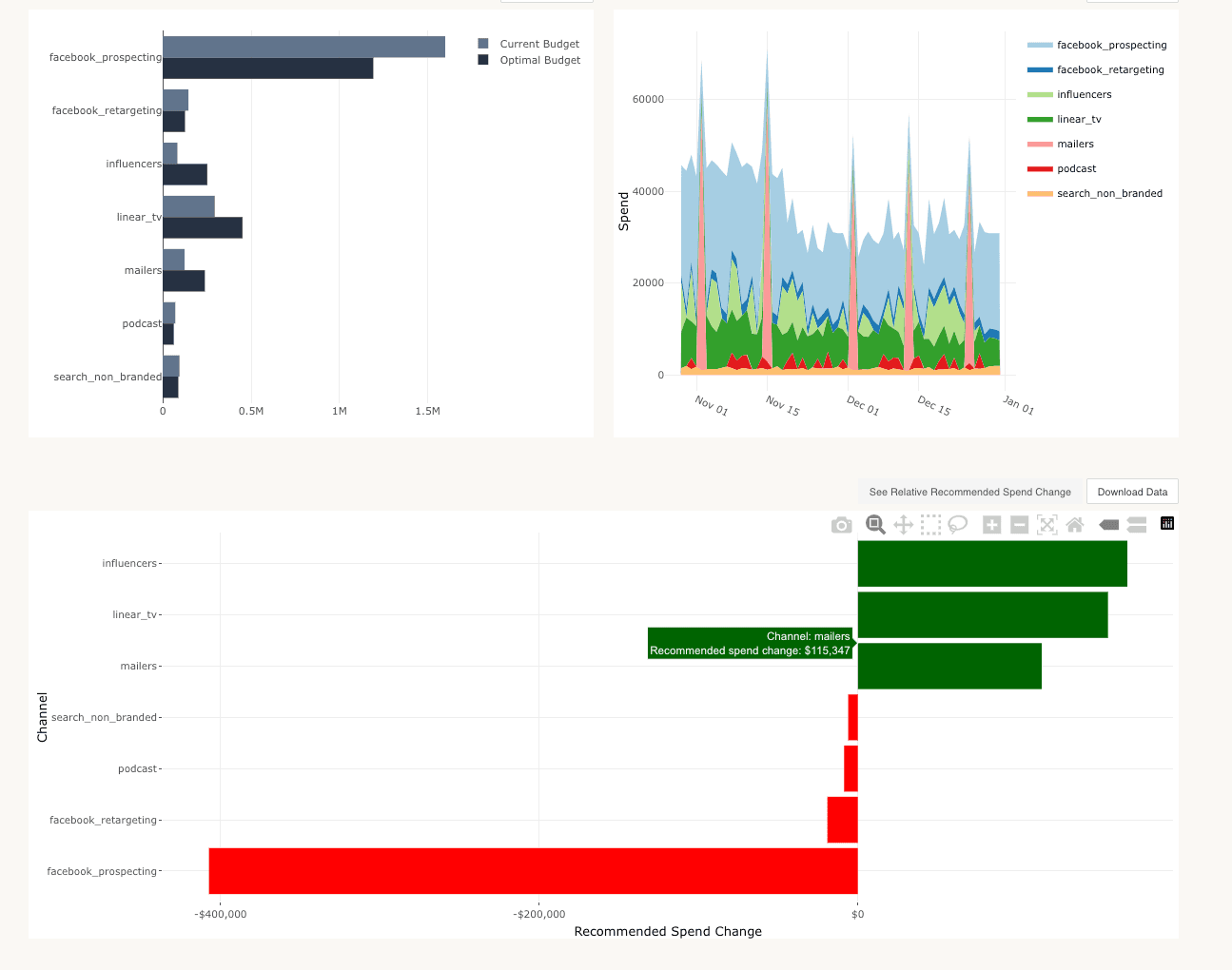
Sample MMM dashboard from Recast
Closing Thoughts
Marketing mix modeling will become an increasingly mainstream part of analytics toolkits to measure marketing channel effectiveness and optimize budgets. MMM does not deal with the data of individual consumers, so it is uniquely insulated as a measurement technique from an evolving privacy environment
At its core, MMM relies on deep historical data spanning all aspects of a business–which makes the data warehouse an essential toolkit for enterprises that need to collect, transform, and activate data for MMM. Hightouch is a leading Data Activation solution, so we make it easy to deliver warehouse data to attribution platforms like Rockerbox. We see the warehouse becoming even more important for marketers as MMM continues to take hold.
If you want to learn more about how to prepare your warehouse data for MMM and sync it to downstream tools, reach out for a demo of Hightouch today.